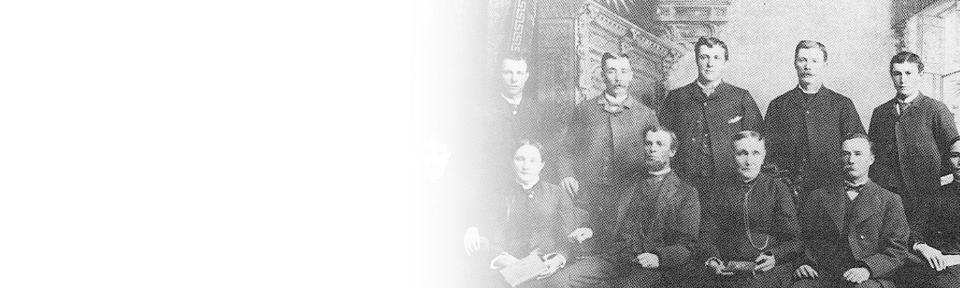
UPDB - Geospatial Services
The Utah Population Database (UPDB) offers comprehensive geospatial consulting services to assist investigators with the following geospatial concentrations: data modeling, data analysis, data linking, data creation, and data visualization. Our geospatial data scientist serves as an investigator of UPDB and a staff scientist, providing a rich knowledge and understanding of the data.
Services include spatial data modeling, geospatial analysis, geospatial familiarity analysis, and environmental exposures studies, service area definitions, point and area measure attribution, study mapping. A few of the most common applications are described below.
Spatial Data Modeling
Spatial data modeling (SDM) is the geographic arrangement of phenomena or identifying the arrangement of geographic phenomena over space and time, revealing geographic patterns within the data. Modeling data spatially can provide ideas about spatial distribution patterns, geospatial event phenomena and resulting outcomes.
Study cohorts and variables can be spatially modeled, including residential location over space and time, environmental exposures, proximity to amenities and services, historical and predictive trend data.
SDM results can guide an investigation to include variables or further information a hypothesis for additional testing.
- Site Analysis: Identify specific geographic locations of interest for studying a disease, exposure, or both.
- Service Area Calculations: Identify the area a defined area around hospitals and clinics for which patients are most likely to visit the clinic.
- Drive Time and Drive Distance Calculations: Identify the driving distance and driving time it takes to get to medical health services.
Geospatial Cluster Analyses
Geospatial cluster analysis is a statistical technique used to identify data spatially and or temporally based on its geographic position. Several different geostatistical methods exist to accomplish this.
- Gravity/Density-based (DBAN): Clusters are identified and defined by proximity to data points within the same dataset.
- Hierarchical: Clusters are identified by their attributes and their proximity to similar or same attribute data point locations.
- Model-based: Clusters are identified within a dataset using a model that will optimize the fit between the data and the model.
- Partition/Attribute-based: Clusters are identified by their attributes.
Environmental Exposure Analysis
Environmental exposure analysis combines environmental data modeling with proximity analysis and statistical analysis. Geospatial exposures include proximity to amenities or medical care, proximity to family, exposure to environmental pollutants such as Toxic Release Inventory (TRI) emissions, roadway emissions, superfund sites, or exposures provided by the investigator. Exposures can be analyzed for individual contact, intergenerational exposure, or transgenerational outcomes.
UPDB has numerous environmental exposure variables that can be linked to individuals or aggregated to government designated area units like census block groups, zip codes, and counties, or natural are units like airsheds or water sheds. Some of our environmental polluting data in UPDB includes:
- Toxic Release Inventory
- Hazardous Waste Facilities
- Superfund sites
- Military Munition Areas (MMA)
- Formerly Used Defense Sites (FUDS)
- Brown Fields
- Active and Inactive Mines
- Leaking Underground Storage Tanks (LUST) Sites
- Roadways
- Landfills
- Land Use and Land Cover
Geospatial Familiarity Analysis
Geospatial familiarity analysis involves identifying multiple generations of family within UPDB and their residential locations over space and time in relation to each other. This type of analysis can be used to identify shared space and time over multiple generations. It can be used to identify environmental contributing factors (cultural, weather and climate, economic conditions, polluting conditions, communicable diseases, generational access to care, etc.) to familiar disease outcomes.
Service Areas
A service area can be defined geographically based on a distance measure to and from a central location or multiple locations. Often investigators are interested in the communities that are directly serviced around a medical clinic(s). Walking distance or driving distance can be used to calculate the area of a community surrounding a clinic with the greatest access. Individuals that have residences within the service area can be identified.
Point and Area Measure Attribution
Point and area measure attribution is the assigning of geospatial data attributes to individual residential point locations for a given time, or to their area level designation, such as zip code or Census area. For example, if a study is interested in any geospatial data that co-occurs at the residential location of a study cohort and the data is available in UPDB or to import into UPDB, the data can be linked to the study cohort in a table as a variable. One example would be Census measures available at the block group level. Many measures exist that can be linked to individual residential locations. If an investigator is interested in the Area Deprivation Index (ADI) for all street address point locations in a study, the data can be linked to the cohort.
Study Mapping
Often study results are interesting and need visual representation. Mapping the results geospatially is a powerful tool for conveying information quickly and effectively. UPDB can map your results for presentations, grants, and publications. We can work directly with you to create design and color styles and templates that meet design guides and requirements.
Contact Us
Utah Population Database Director
Nicola Camp, PhD
Utah Population Database Operations Manager
Dinah Busico, MPA
Governance
HCI Senior Director Oversight
Tracy Onega, PhD, MS
Faculty Advisory Committee Chair
Anne Kirchhoff PhD
Faculty Advisory Committee Members
Djin Tay, PhD
Britton Trabert, PhD
Joshua Schiffman, MD
Arabella Young, PhD
Srinivas Tantravahi, MBBS, MRCP
Amanda Bakian, PhD
David Curtis, PhD
Mick Jurynec, PhD
Joemy Ramsay, PhD
Lauren Theilen, MD
Marcus Pezzolesi, PhD